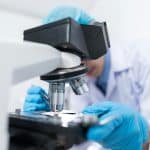
Steps to Integrate AI into Medical Data Administration
November 16, 2024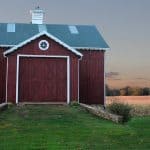
Converting Barns to Homes: Georgia’s Unique Properties
January 10, 2025Understanding Human Feelings
AI systems can now read non-verbal expressions and decipher emotions like anger, fear, sadness and happiness with remarkable accuracy. This breakthrough allows businesses to improve customer service and foster more empathetic relationships.
Businesses are capitalizing on emotional AI in various industries such as healthcare, business marketing and gaming. However, it’s essential to navigate ethical considerations with care and foresight.
Voice Recognition
As technology evolves, human-machine interactions are becoming increasingly complex. Voice AI is a prime example of this shift, as it enables people to communicate with and control devices through spoken commands. Its evolution from rudimentary speech recognition systems to the sophisticated conversational interfaces of contemporary smart devices is a testament to human ingenuity and the relentless pursuit to make technology more intuitive and accessible.
A key component of Voice AI is natural language processing (NLP), which enables verbal interfaces to understand context, tone, and intent. Whether they are asking for directions or searching the internet, users want to be understood and feel connected to their technology. NLP makes this possible by using machine learning to train systems on a large volume of data and continuously refine and improve their performance.
NLP is also used in voice automation to provide personalized and contextual responses to user requests. For example, when calling a customer service center, users are often greeted with a menu that asks them to select an option based on their reason for calling. These automated systems use NLP to detect their mood and choose an appropriate response from a library of options. This level of personalization helps to reduce call times and increase customer satisfaction.
Another area in which NLP is used in voice AI is for medical applications, such as dictating notes or transcribing doctor’s visits. This allows healthcare professionals to focus on patients, rather than the administrative tasks associated with documenting meetings and depositions. In addition to NLP, medical professionals are leveraging other technologies that can help them better manage their workloads and optimize patient care.
These technologies include acoustic modeling, which analyzes the sounds of spoken words to identify their structure and meaning. This is then combined with linguistic modeling, which identifies the grammar and syntax behind spoken phrases to transform them into readable, actionable information. The combination of these technologies enables Voice AI to transcribe audio, perform simple searches, and understand natural speech.
Many consumers have come to believe that they can develop an emotional connection with their voice assistants. In fact, according to a 2022 study, one-third of U.S. adults agree that they love their AI. This sentiment may reflect a deeper societal need for human connection, as evidenced by the rise of loneliness as a silent epidemic.
Emotion Recognition
A key aspect of human AI is emotion recognition, which involves understanding a person’s emotional state based on visual cues. Emotion recognition can be used in a wide range of applications, from facial expression analysis to human-machine interface design and humanoid robot development.
While people still have the advantage in reading emotions, machines are becoming better at this task as they learn to analyze vast amounts of data, says MIT Sloan Professor Erik Brynjolfsson. For example, they can now recognize subtle differences in vocal inflections that correlate with stress or anger, and pick up micro-expressions on a face that might happen too fast for a human to perceive.
In the early 2000s, researchers in Sweden created a database of images that displayed posed faces with various emotional states. This data set helped to train the first wave of emotion recognition systems. These systems used deep learning to identify six basic emotions: fear, surprise, anger, sadness, happiness, and disgust.
Since then, the technology has improved considerably, thanks to advances in neural network architectures and computing power. In particular, Variational Autoencoders (VAEs) can now compress a large volume of input data – including body movements, facial expressions, and voice tones – into a small number of essential traits that can be recognized by an algorithm.
However, even as this technology improves, it can have unintended consequences. Job applicants may be judged unfairly if their facial expressions appear angry, students are flagged if they seem to be stressed, and customers may be questioned if they look like shoplifters. Some privacy and human rights advocates argue that these systems are invasive and rely on questionable methodologies.
Emotion recognition also generates a lot of data, which can include personal information such as health and location. This can raise concerns about privacy and security, especially for large-scale IoT systems that are incorporating emotion recognition into their operations. It is important to develop a privacy framework that can help to protect these systems and to ensure they are only used in appropriate contexts.
Emotional Learning
As human AI develops further, the ability to interpret and respond to emotional cues is enabling it to form deeper connections with users. This “emotional intelligence” is revolutionizing user experiences, creating opportunities for improved communication and collaboration between humans and AI systems. However, as with any new technology, the evolution of human-AI relationships raises several ethical questions that need to be addressed.
In an age where many people rely on their computers for daily tasks, companionship with AI bridges the gap to challenge traditional notions of personal and professional identity. In some cases, these relationships may even begin to compete with real-life social and romantic connections. This burgeoning pseudo-intimacy raises concerns that artificial companions could undermine human psychological needs and lead to unhealthy patterns of behavior.
Emotional learning in AI refers to the ability of a computer system to perceive and understand human emotions through non-verbal cues, such as facial expressions or tone of voice. The emergence of this type of intelligence has facilitated a variety of applications for improving AI systems, including enhancing user experience and increasing trust. Despite the benefits of emotional learning, it is crucial that designers of human-AI interactions are aware of potential ethical implications.
The burgeoning popularity of human-AI relationships highlights the need for research into social and emotional interaction design. A growing body of evidence supports the idea that certain social and emotional interactions are essential to human cognitive and emotional well-being, but there is a gap in knowledge about how to design these interactions effectively.
A critical step in this process is understanding the relationship between rapport, trust, user engagement, empathy and anthropomorphization. These interconnected themes converge in AI systems to enhance user satisfaction and commitment. Moreover, understanding these socio-emotional dynamics will help designers of human-AI systems develop more sophisticated and meaningful interactions that are ethically informed.
Rapport is a harmonious relationship underpinned by mutual understanding and empathetic engagement between interacting entities (Gremler and Gwinner, 2008). In human-AI interactions, rapport directly influences the effectiveness of communication and trust, which in turn influences user commitment.
Empathy is a key component of human-AI interactions, and it can be enhanced through anthropomorphization, a process whereby an object or entity is endowed with human-like characteristics that make it more relatable to the user. The use of anthropomorphization in human-AI interactions allows AI systems to convey greater empathy, which contributes to higher levels of user satisfaction and a more positive overall experience.
Emotional Intelligence
The rise of emotional AI has profoundly changed the way humans interact with and connect to each other. It’s also changed the dynamics of human-AI interactions and impacted how users engage with their devices. For example, some people develop an attachment to their AI companions, forming a bond that transcends traditional definitions of love and relationships. This phenomenon challenges popular narratives that conflate human evolution with the fate of the human species and opens the door to new perspectives on the impact of AI on the broader society.
Emotional intelligence enables algorithms to respond more empathetically to user needs and emotions. It’s based on the ability to recognize and interpret emotions from multiple sources, including text, voice, facial expressions, and physiological signals. This technology is critical for enhancing customer service, providing personalized healthcare, and boosting productivity in many sectors.
Affective computing is a burgeoning field that aims to analyze user behavior and emotion through technology like artificial intelligence, computer vision, natural language processing, and other techniques. Affective computing is an interdisciplinary field, encompassing aspects of psychology, philosophy, sociology, and computer science. It explores the effects of emerging technologies on human behavior, focusing on emotions, perception, and cognition.
ML algorithms use data from various sources to recognize patterns of emotions and determine whether a person is in a positive, negative, or neutral mood. This information is then used to modify the behavior of the algorithm. It’s an iterative process, with the algorithm improving over time and becoming more accurate with each interaction. This process is referred to as deep learning, as the algorithms learn from each iteration and improve with each one.
The goal of integrating emotion AI is to create more empathetic and effective interactions for users across various industries. For example, Cogito uses its emotional AI to provide real-time feedback to call center agents and enable them to deliver a better customer experience. Moreover, the technology enables healthcare providers to detect an individual’s emotional state and deliver tailored, empathetic care. Lastly, emotional AI helps businesses boost customer service and drive sales by identifying the underlying emotions in conversations.